New research from U.S. Naval Research Laboratory (NRL) researchers delivers a novel contribution to fiber optics computing. Titled “Fiber optic computing using distributed feedback,” the paper published in Communications Physics journal brings the Navy one step closer to faster, more efficient computing technologies.
Optical computing uses the properties of light such as its speed and ability to carry large amounts of data in order to process information more efficiently than traditional electronic computers.
In collaboration with Sandia National Laboratories and the University of Central Florida, NRL is aiming to increase processing speeds, reduce energy consumption, and enable new applications in fields such as data processing, telecommunications, and artificial intelligence.
“This paper marks a significant advancement in optical computing,” said Brandon Redding, Ph.D., a research physicist from the NRL Optical Sciences Division. “It is the first to employ distributed feedback in optical fiber, combining temporal encoding with low-loss, partially reflective fiber. Our approach offers scalability to process multiple neurons simultaneously, along with high-speed performance and a compact, lightweight, and power-efficient design, as the entire system is fiber-coupled and does not require free-space optics.”
The Navy is rapidly adopting machine learning algorithms for a wide range of applications. Many of these applications are time and energy-sensitive; for instance, image or target recognition tasks where objects require identification in real time.
“Many of these applications involve forward-deployed, often autonomous platforms with limited power availability,” Redding said. “We intend to use analog photonics, which has fundamentally different energy scaling than Von Neumann-based digital electronics, to perform these machine learning tasks with lower power consumption and with lower latency. In the current paper, we performed an energy consumption analysis showing the potential for 100â1000 times lower power consumption than a GPU depending on the problem size.”
This research shows how optics can be used to conduct valuable computing tasks using passive random projections, in this case, non-linear random convolutions. This is counter to how most machine learning works, which typically requires extensive training to set the weights of a neural network.
“Instead, we show that random weights can still perform useful computing tasks,” Redding said. “This is significant because we can apply random weights very efficiently in the optical domain simply by scattering light off of a rough surface, or as we show in this paper, scattering light off non-uniformities in an optical fiber.”
In traditional digital electronics-based computers, there wouldn’t be much advantage to doing this because every multiplication operation is just as expensive in terms of time and energy, whether multiplying by a random number or by a value carefully selected through training.
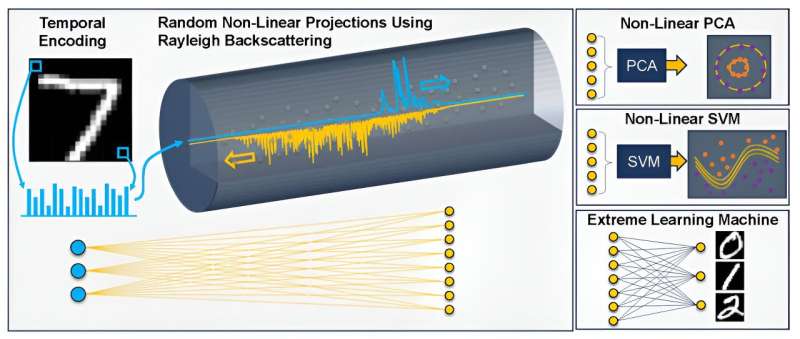
“This implies that in the optical domain, we may want to design our neural network architectures differently to take advantage of the unique features of opticsâsome things are easier to do in optics and some things are harder. Therefore, simply porting the same neural network architecture that was optimized for digital electronics implementations may not be the ideal solution in the optical domain,” Redding said.
A more subtle feature of NRL’s fiber platform is performing convolutions, similar to a convolutional neural network (CNN), a rarity for an optical computing platform. Convolutions are very powerful for tasks like image processing, which led to the widespread use of CNNs within the Department of Defense image processing applications.
“The Navy payoff is implementing machine learning algorithms faster, reducing the delay before we arrive at an answer,” said Joseph Murray, Ph.D., a research physicist from the NRL Optical Sciences Division. “We are also exploring applying these algorithms directly on analog data without requiring intermediate digitization and storage, which could have a significant benefit when processing high bandwidth data that is difficult to record and analyze in real-time, such as high-resolution image data or RF data for electronic warfare applications.”
The research, both theoretical and experimental, is concerned with discovering and understanding the basic physical principles and mechanisms involved in optical devices and materials.
“The current paper is the proof-of-principle that we can do useful computing with these fixed, random optical projections, as tested on benchmark tasks like image recognition of handwritten digits,” said Joseph Hart, Ph.D., a research physicist from the NRL Optical Sciences Division. “We also tested this on a SONAR dataset task to show how this platform can discriminate between SONAR signatures from rocks versus underwater mines as a more Navy-specific application.”
The Optical Sciences Division carries out a variety of research, development, and application-oriented activities in the generation, propagation, detection, and use of radiation in the wavelength region between near-ultraviolet and far-infrared wavelengths. The Division serves the Laboratory and the Navy as a consulting body of experts in optical sciences.
More information:
Brandon Redding et al, Fiber optic computing using distributed feedback, Communications Physics (2024). DOI: 10.1038/s42005-024-01549-1
Citation:
Physicists explore fiber optic computing using distributed feedback (2024, March 11)
retrieved 11 March 2024
from
This document is subject to copyright. Apart from any fair dealing for the purpose of private study or research, no
part may be reproduced without the written permission. The content is provided for information purposes only.