Machine learning-based models that can autonomously generate various types of content have become increasingly advanced over the past few years. These frameworks have opened new possibilities for filmmaking and for compiling datasets to train robotics algorithms.
While some existing models can generate realistic or artistic images based on text descriptions, developing AI that can generate videos of moving human figures based on human instructions has so far proved more challenging. In a paper pre-published on the server arXiv and presented at The IEEE/CVF Conference on Computer Vision and Pattern Recognition 2024, researchers at Beijing Institute of Technology, BIGAI, and Peking University introduce a promising new framework that can effectively tackle this task.
“Early experiments in our previous work, HUMANIZE, indicated that a two-stage framework could enhance language-guided human motion generation in 3D scenes, by decomposing the task into scene grounding and conditional motion generation,” Yixin Zhu, co-author of the paper, told Tech Xplore.
“Some works in robotics have also demonstrated the positive impact of affordance on the model’s generalization ability, which inspires us to employ scene affordance as an intermediate representation for this complex task.”
The new framework introduced by Zhu and his colleagues builds on a generative model they introduced a few years ago, called HUMANIZE. The researchers set out to improve this model’s ability to generalize well across new problems, for instance creating realistic motions in response to the prompt “lie down on the floor,” after learning to effectively generate a “lie down on the bed” motion.
“Our method unfolds in two stages: an Affordance Diffusion Model (ADM) for affordance map prediction and an Affordance-to-Motion Diffusion Model (AMDM) for generating human motion from the description and pre-produced affordance,” Siyuan Huang, co-author of the paper, explained.
“By utilizing affordance maps derived from the distance field between human skeleton joints and scene surfaces, our model effectively links 3D scene grounding and conditional motion generation inherent in this task.”
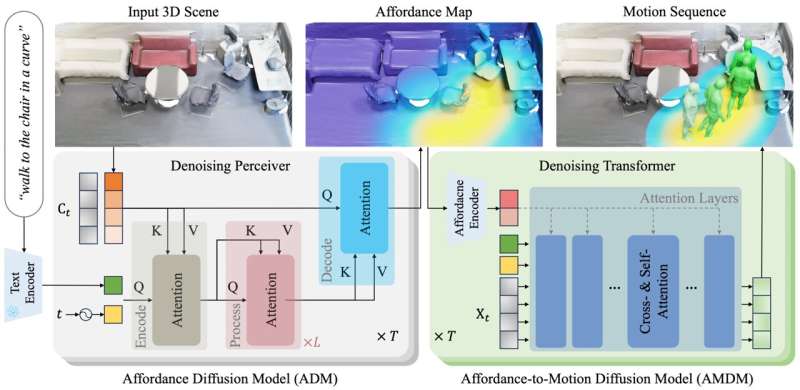
The team’s new framework has various notable advantages over previously introduced approaches for language-guided human motion generation. First, the representations it relies on clearly delineate the region associated with a user’s descriptions/prompts. This improves its 3D grounding capabilities, allowing it to create convincing motions with limited training data.
“The maps utilized by our model also offer a deep understanding of the geometric interplay between scenes and motions, aiding its generalization across diverse scene geometries,” Wei Liang, co-author of the paper, said. “The key contribution of our work lies in leveraging explicit scene affordance representation to facilitate language-guided human motion generation in 3D scenes.”
This study by Zhu and his colleagues demonstrates the potential of conditional motion generation models that integrate scene affordances and representations. The team hopes that their model and its underlying approach will spark innovation within the generative AI research community.
The new model they developed could soon be perfected further and applied to various real-world problems. For instance, it could be used to produce realistic animated films using AI or to generate realistic synthetic training data for robotics applications.
“Our future research will focus on addressing data scarcity through improved collection and annotation strategies for human-scene interaction data,” Zhu added. “We will also enhance the inference efficiency of our diffusion model to bolster its practical applicability.”
More information:
Zan Wang et al, Move as You Say, Interact as You Can: Language-guided Human Motion Generation with Scene Affordance, arXiv (2024). DOI: 10.48550/arxiv.2403.18036
© 2024 Science X Network
Citation:
A new framework to generate human motions from language prompts (2024, April 23)
retrieved 23 April 2024
from
This document is subject to copyright. Apart from any fair dealing for the purpose of private study or research, no
part may be reproduced without the written permission. The content is provided for information purposes only.